|
|
|
|
Abstract
Recent large machine learning models have achieved impressive performance while showing some shared similarities with human biology. In this work, we pose the question of whether big vision language models, specifically, CLIP, are able to capture optical illusion which is tightened to human biology and perception. We measure the effect by presenting a variety of illusions in the form of images and texts to CLIP and observing how the model’s classification score changes under different conditions of the illusion. Our results show that CLIP is able to capture different types of illusions like lightness illusion and geometrical illusion. We also propose a way to calibrate CLIP score to reduce biases.
Vasarely Illusion
Non-Calibrate:
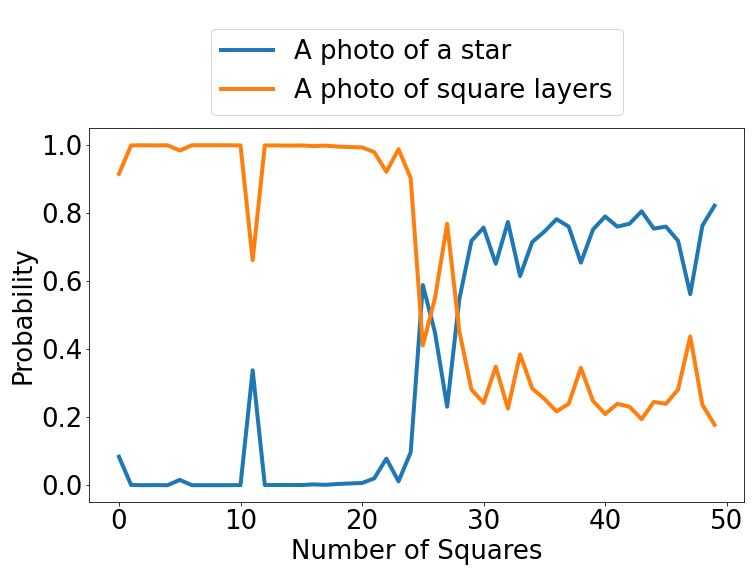
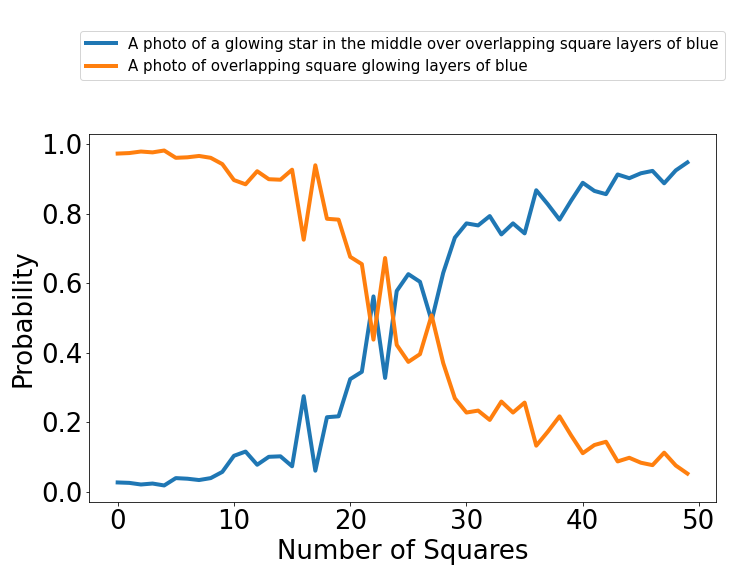
Calibrate:
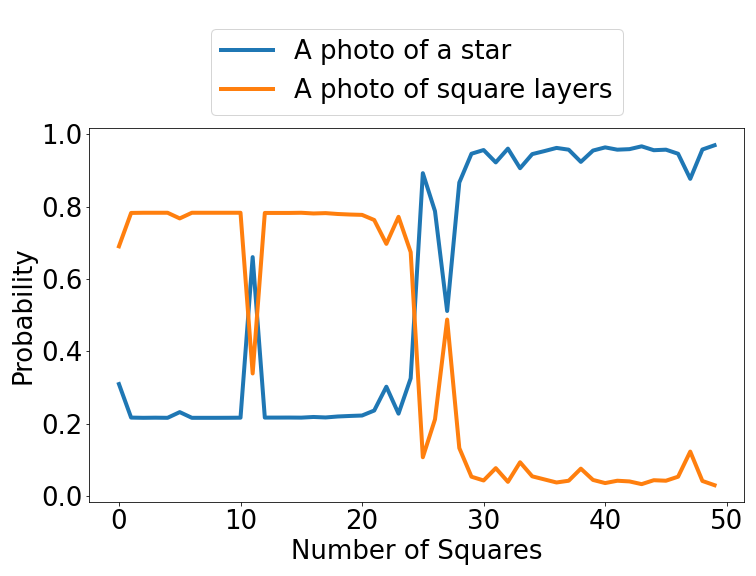
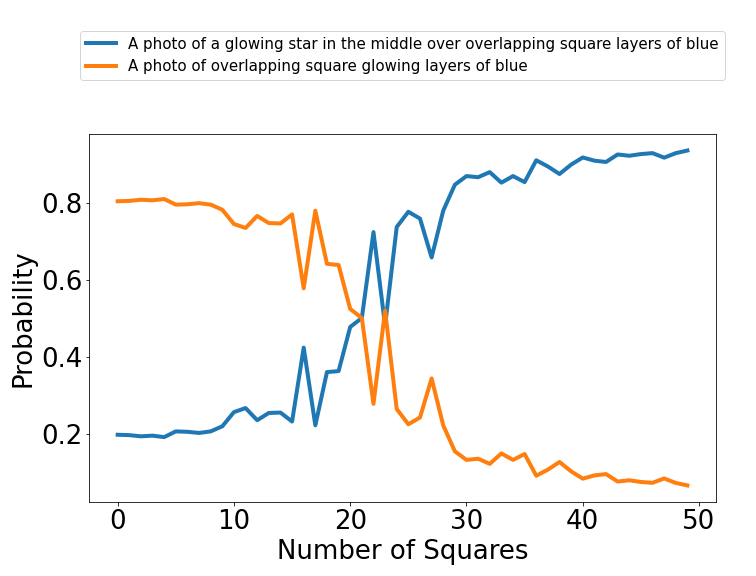
Ponzo Illusion
Non-Calibrate:
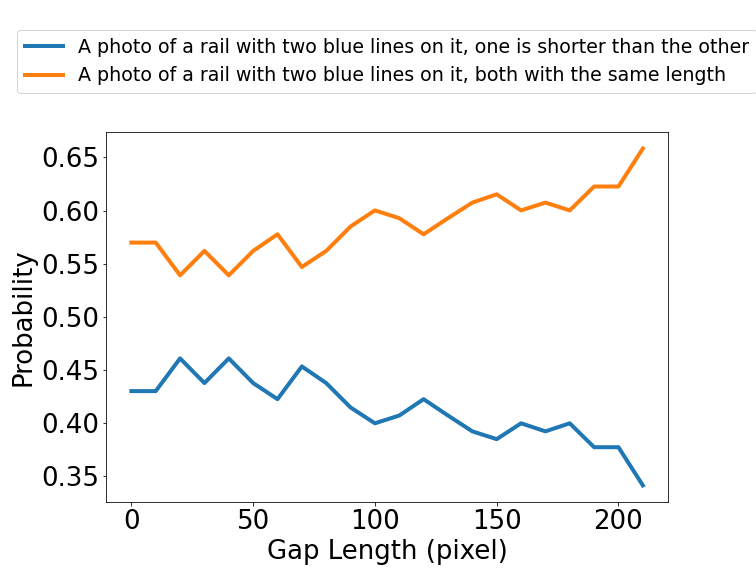
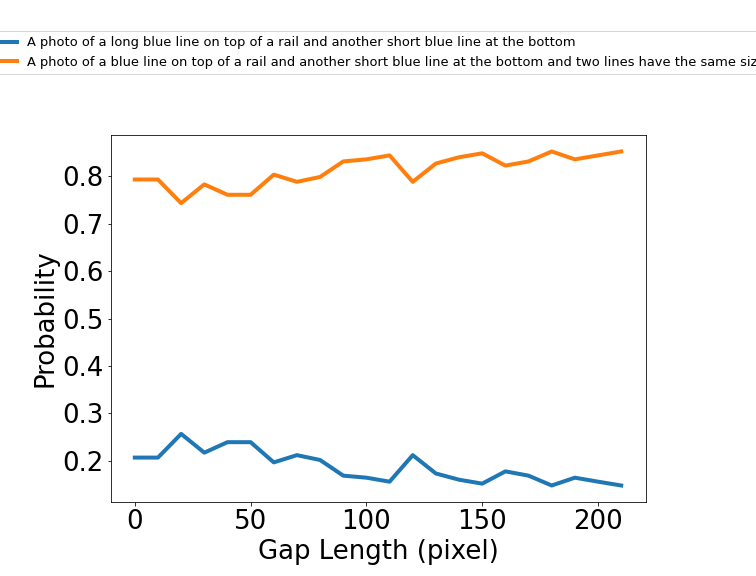
Calibrate:
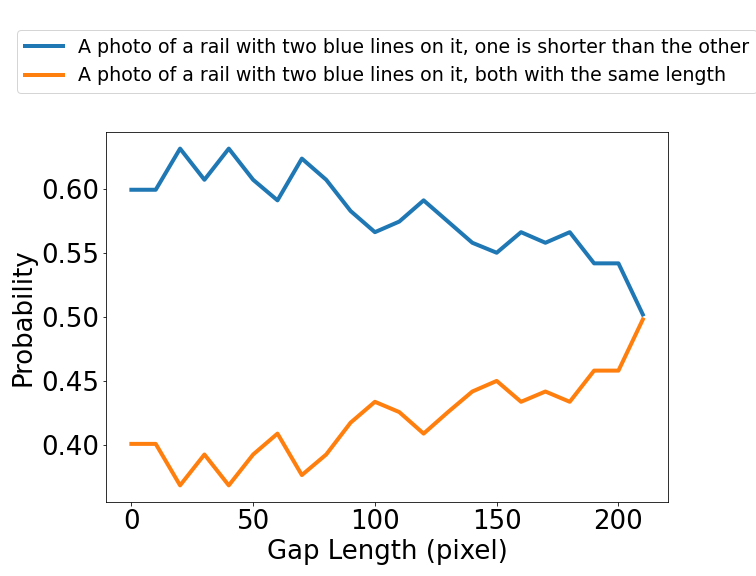
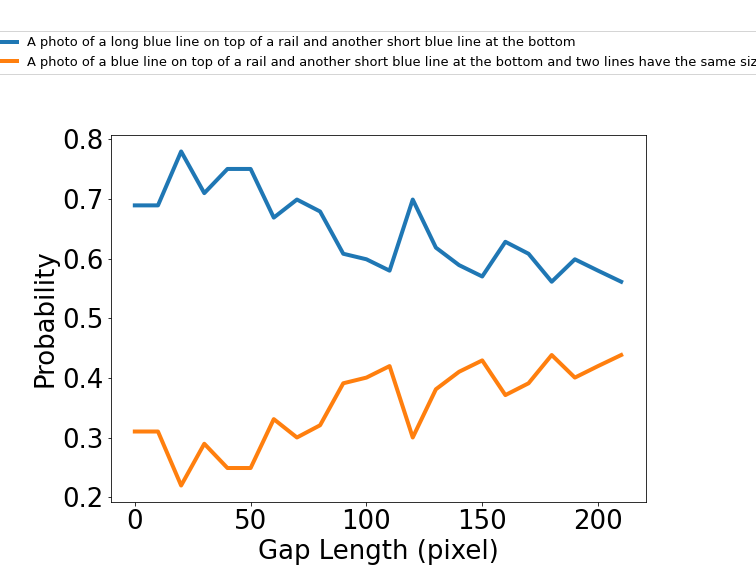
Simultaneous Contrast Illusion
Non-Calibrate:
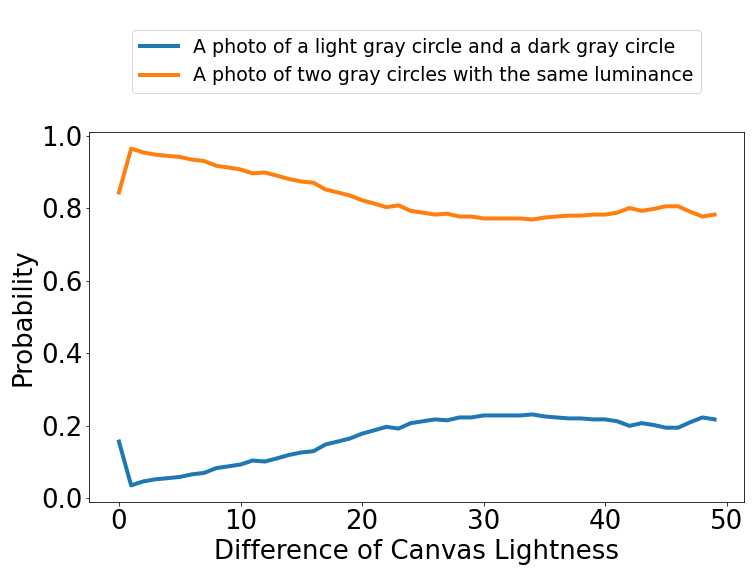
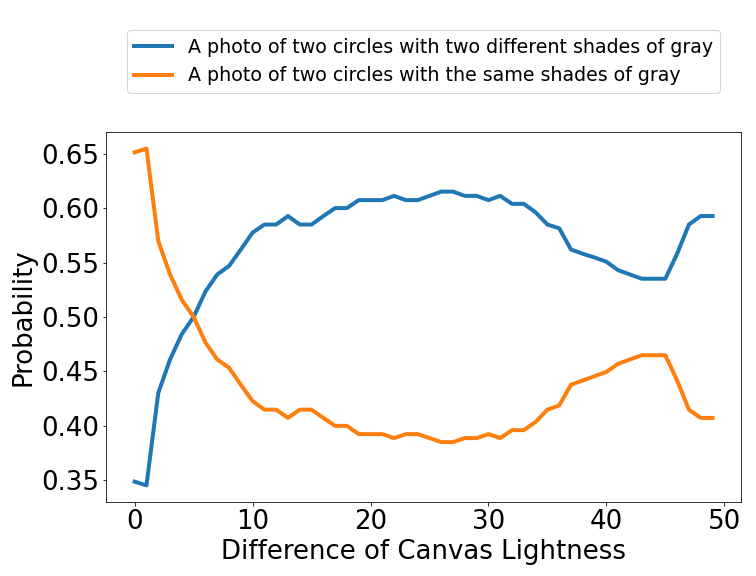
Calibrate:
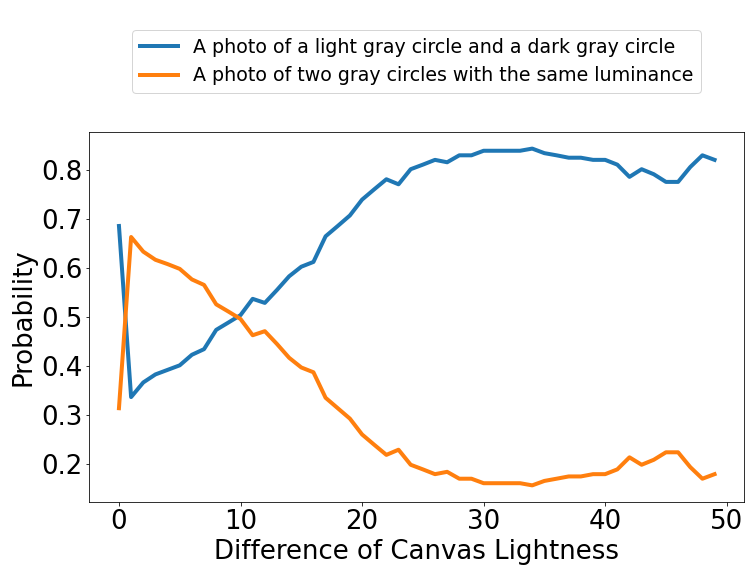
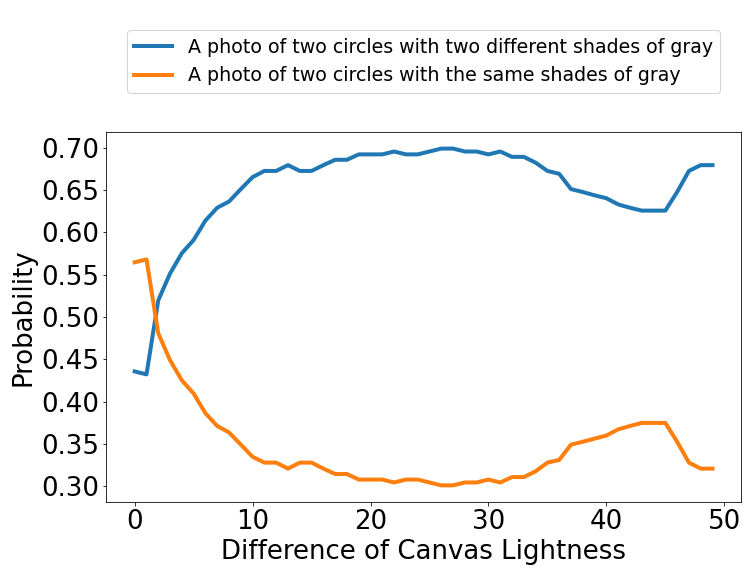